by Damiano Gasparotto and Ivan Masnari, Junior Data Scientist and Software Developer in Excellence Innovation
Generative artificial intelligence is making great strides, offering more and more surprising results every week. The most recent and powerful models can process answers based on a very broad context, even comparable to a 500-page book, while others (the “multimodal”) are able to understand different types of input such as images, documents and datasets.
However, most models available on the market are “generalists”: trained on a huge variety of data, but without specializing in any one task in detail. When you need to have very specific answers, the output is not always satisfactory or consistent, even using the most powerful models. Furthermore, if “open-weights” or non-top-of-the-range models are used, the quality and stability of the responses tends to deteriorate significantly.
To delve deeper into the topic, we wanted to analyze two aspects:
– Would the best market models be able to pass the OCF exam to qualify as a Financial Advisor?
– Is it possible to take a much smaller “open-weights” model and train it to achieve the same goal?
The AI at the OCF exam test
After having analyzed the financial performance of the main artificial intelligence models, we continued the experiments with the aim of evaluating the effectiveness of training smaller and open-weight models, the so-called “fine-tuning”. As an area of specialization we have selected the OCF evaluation test, the exam necessary for registration in the register of Financial Consultants in Italy.
The test included 60 multiple choice questions on the five subjects included in the official exam. Each test was repeated five times for each model, to evaluate not only the correctness of the answers, but also their consistency.
The models we tested were:
Market models:
* Claude Sonnet 3.5 (latest model from Anthropic)
* GPT-4o (latest model from OpenAI)
* GPT-3.5 turbo (OpenAI model used in the free version of ChatGPT)
Small open-weight models:
* Llama 3-8B basic version
* Llama 3-8B with our fine-tuning
How to improve the competence of a model
There are two fundamental techniques for improving the quality and accuracy of responses
1. Prompt engineering: consists of formulating questions or instructions (the “prompts”) in such a way as to guide the model towards more accurate and relevant answers. (we have explored this aspect in depth in a previous article https://gruppoexcellence.com/2024/03/29/ importance-prompt-engineering-guidare-comportamento-modelli-ai-generativa/ ).
2. Fine-tuning: a more advanced process that “re-trains” the model on sector-specific data, transforming it from a generalist to a specialist.
Fine-tuning is a partial training technique that allows you to obtain a model specialized in a specific task. It can be applied to all models and with different depth levels. A particularly interesting and increasingly adopted solution concerns the fine-tuning of small open-weight models, which potentially allows them to improve their performance without requiring enormous computing capacity.
How we trained the model
For the training experiment we chose Llama 3-8B as the base model, the reduced version of Meta’s latest open-weights model, known for its good performance in relation to its small size, and we developed a dataset of 5000 questions /answers, covering the five main areas of the OCF test:
* Financial market and intermediary law
* Social security and insurance law
* Private law and commercial law
* Tax law for the financial market
* Economics and Mathematics of the financial market
The fine-tuning process applied to Llama 3-8B consisted of a “surface retraining”, aimed at creating a new model that retains the predictive capabilities of the base model, but is specialized in the five areas of the OCF test. This type of fine-tuning does not modify all the parameters, but involves only the main ones (between 1% and 10% of the total).
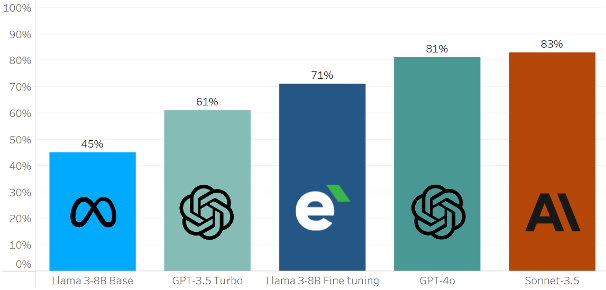
Exam results
Put to the test, the market models obtain the best results, which also confirm the indications we obtained in our previous experiment: in the financial sector, Anthropic models seem to perform slightly better than OpenAI models.
But above all, the test results confirmed the potential of fine-tuning. While the basic Llama 3-8B model does not provide satisfactory results, with our initial fine-tuning it showed a significant improvement, with the percentage of correct answers rising from 45% to 71%.
Comparing the models, the Llama 3-8B model trained with our fine-tuning exceeded the performance of the GPT-3.5 Turbo (61%), and came remarkably close to the results of the most powerful models on the market: GPT-4o ( 81%) and Claude 3.5 Sonnet (83%).